One of the central themes during Snowflake Summit 2023 was bringing Generative AI and Large Language Models to your Data. According to Snowflake’s CEO it’s just like they removed all letters from the alphabet and just left the A and the I there. According to him it could also be a drinking game; take a shot every time someone mentions AI.
What is Generative AI?
Generative AI refers to a branch of artificial intelligence (AI) focused on creating models and algorithms that have the ability to generate new and original content. Unlike traditional AI models that are based on pre-defined rules or patterns, generative AI models are designed to learn the patterns and structure of their input training data and then generate new data that has similar characteristics. Notable generative AI systems include ChatGPT, DALL-E, and Bard
Generative AI has many practical uses, such as creating new product designs, optimizing business processes, generating branded images for ads, developing content ideas based on SEO keywords, writing shareable summaries for long-form articles, and even translating advertisements. It can also be used to create custom generative AI models for advanced services, including chatbots, search, and summarization.
Coöperations
Snowflake recently announced several partnerships and acquisitions in that area.
- NVIDIA
- Neeva
- Applica
NVIDIA
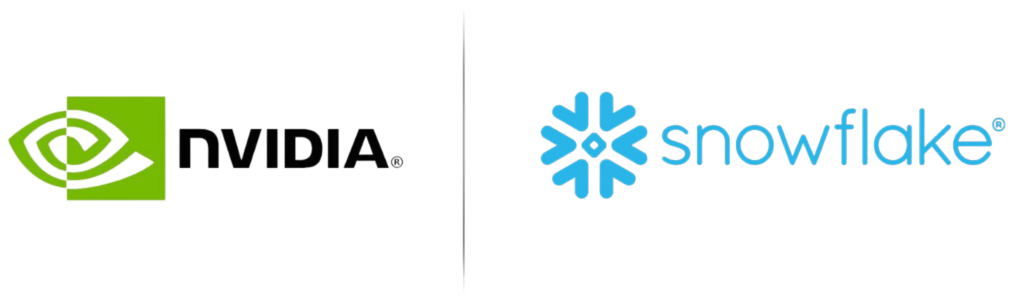
NVIDIA and Snowflake recently announced a partnership to bring accelerated computing to the Data Cloud with the new Snowpark Container Services, a runtime for developers to manage and deploy containerized workload. By integrating the capabilities of GPUs and AI into the Snowflake platform, customers can enhance ML performance and efficiently fine-tune LLMs. They achieve this by leveraging the NVIDIA AI Enterprise software suite on the secure and governed Snowflake platform.
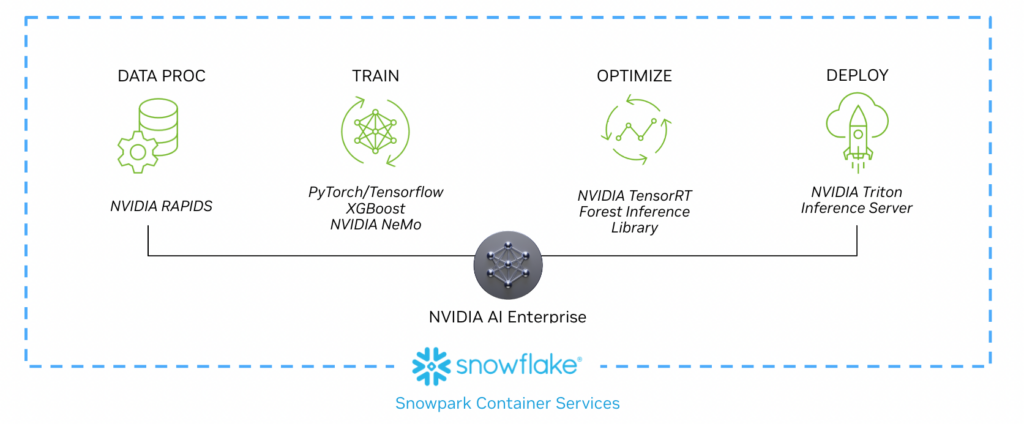
The partnership will provide businesses of all sizes with an accelerated path to create customized generative AI applications using their own proprietary data, all securely within the Snowflake Data Cloud. With the NVIDIA NeMo platform for developing large language models (LLMs) and NVIDIA GPU-accelerated computing, Snowflake will enable enterprises to use data in their Snowflake accounts to make custom LLMs for advanced generative AI services, including chatbots, search, and summarization. Through this partnership Snowflake’s users are enabled to build custom AI models using their internal data, which is a big deal for businesses that want to take advantage of LLMs and generative AI and need to get specific, company-centric answers to their own queries.
Together, NVIDIA and Snowflake will create an AI factory that helps enterprises turn their own valuable data into custom generative AI models to power groundbreaking new applications.
Neeva
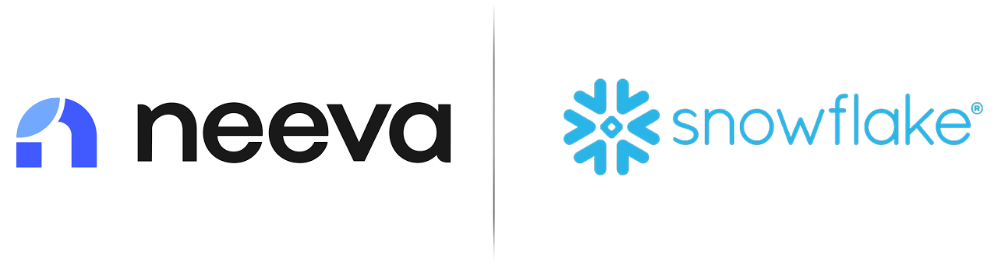
Last may 2023, Snowflake acquired Neeva. The acquisition will enable Snowflake users and application developers to build rich search-enabled and conversational experiences, and will allow Snowflake’s users to build custom AI models using their internal data. They will be able to discover precisely the right data point, data asset, or data insight to maximize the value of data.
It is not yet clear how Snowflake will integrate Neeva’s technology into its data cloud platform. However, Snowflake plans to infuse and leverage Neeva’s search capabilities across the Snowflake Data Cloud, which suggests that Neeva’s AI-driven search technology will be integrated into Snowflake’s platform to enhance its search capabilities
Neeva Co-Founder; Sridhar Ramaswamy shares thoughts on the future of Generative AI and his company’s acquisition by Snowflake.
Applica
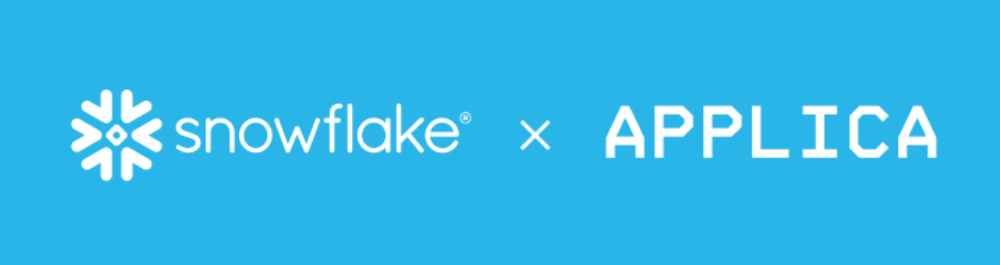
In august 2022, Snowflake acquired Applica; a Poland-based AI platform for document understanding. The acquisition was aimed at helping Snowflake customers more easily leverage unstructured data in the Snowflake Data Cloud.
Snowflake customers use the Data Cloud to bring together all types of data to support a variety of deployment patterns, while ensuring fast, governed data access at scale. Earlier in 2022, Snowflake added support for unstructured data in the Snowflake Data Cloud, featuring built-in capabilities to store, manage, govern, share, and process unstructured data with the same performance, concurrency, and scale as structured and semi-structured data.
Takeaways
I attended various Keynotes where Generative AI was a topic. I was glad to hear that the focus was not only on how great Generative AI is. AI Technology is an enabler. It’s another tool in our toolbox, which opens new use cases. Fortunately AI has become natural, you do not have to speak 1s and 0s. Unstructured data and LLMs work really well together.
A Data Strategy is key for successful AI initiatives. This ensures access to relevant and high-quality data, address ethical considerations, enhance data security, support iterative improvement, optimize resource utilization, and align with business objectives.
- Data Availability: Generative AI models require large amounts of high-quality data to learn and generate meaningful outputs. A data strategy helps identify and acquire the necessary data sources, ensuring a sufficient and diverse dataset for training the models.
- Data Quality: To achieve accurate and reliable results, data used in generative AI must be clean, labeled, and representative of the desired outcomes. A data strategy includes data preprocessing and quality assurance measures to enhance the reliability and effectiveness of the generative AI models.
- Ethical Considerations: Generative AI has the potential to generate content that may be controversial, biased, or infringing on privacy rights. A data strategy helps address ethical concerns by defining data collection, usage, and governance policies that align with legal and ethical guidelines.
- Data Security: Handling large volumes of data for generative AI models requires robust security measures to protect sensitive information and prevent unauthorized access. A data strategy outlines security protocols, data storage, access controls, and compliance measures to ensure data privacy and security.
- Iterative Improvement: Generative AI models benefit from iterative training and continuous improvement. A data strategy incorporates mechanisms for collecting user feedback, monitoring model performance, and incorporating new data to refine and enhance the generative AI outputs over time.
- Resource Optimization: Generative AI models can be computationally intensive and resource-consuming. A data strategy helps optimize resource allocation by identifying data subsets, prioritizing specific data attributes, or using techniques like transfer learning to leverage pre-existing models and datasets efficiently.
- Business Alignment: A data strategy aligns generative AI initiatives with business goals and objectives. It defines the intended use cases, target audience, and expected outcomes to ensure that generative AI efforts contribute to the organization’s overall strategy and deliver tangible value.
State of Generative AI 2024
The innovations in Generative AI go very fast. Here are a few thoughts about of the State of Gen AI in 2024
- Multimodality capibility – Multimodality refers to the integration and processing of multiple modes or types of data within an artificial intelligence (AI) system. In the context of AI, modalities refer to different types of information, such as text, images, audio, video, or sensor data.
- Computer Vision – Computer Vision refers to the field of artificial intelligence and computer science that focuses on enabling computers to gain a visual understanding of the world. Where current Gen AI focus on text, the future lies in image-, and video transformers (80% of the world’s data)
- Explainability & Trustworthiness – Explainability refers to the ability to understand and explain how and why a generative AI model generates a particular output. It is essential for ensuring transparency, accountability, and building trust in the generated results. Trustworthiness is crucial to ensure that the generated content is reliable, unbiased, and does not propagate harmful or misleading information
Part of is this blog is generated with ChatGPT and Perplexity.
Till next time.
Director Data & AI at Pong and Snowflake Data Superhero. Online better known as; DaAnalytics.